前言
之前書寫了使用pytorch進(jìn)行短文本分類,其中的數(shù)據(jù)處理方式比較簡單粗暴。自然語言處理領(lǐng)域包含很多任務(wù),很多的數(shù)據(jù)向之前那樣處理的話未免有點(diǎn)繁瑣和耗時。在pytorch中眾所周知的數(shù)據(jù)處理包是處理圖片的torchvision,而處理文本的少有提及,快速處理文本數(shù)據(jù)的包也是有的,那就是torchtext[1]。下面還是結(jié)合上一個案例:【深度學(xué)習(xí)】textCNN論文與原理——短文本分類(基于pytorch)[2],使用torchtext進(jìn)行文本數(shù)據(jù)預(yù)處理,然后再使用torchtext進(jìn)行模型分類。
關(guān)于torchtext的基本使用除了可以參考官方文檔,也可以看看這篇文章:TorchText用法示例及完整代碼[3]。
下面就開始看看該如何進(jìn)行處理吧。
1 數(shù)據(jù)處理
首先導(dǎo)入包:
from torchtext import data
我們處理的語料中,主要涉及兩個內(nèi)容:文本,文本對應(yīng)的類別。下面使用torchtext構(gòu)建這兩個字段:
# 文本內(nèi)容,使用自定義的分詞方法,將內(nèi)容轉(zhuǎn)換為小寫,設(shè)置最大長度等 TEXT = data.Field(tokenize=utils.en_seg, lower=True, fix_length=config.MAX_SENTENCE_SIZE, batch_first=True) # 文本對應(yīng)的標(biāo)簽 LABEL = data.LabelField(dtype=torch.float)
其中的一些參數(shù)在一個config.py文件中,如下:
# 模型相關(guān)參數(shù) RANDOM_SEED = 1000 # 隨機(jī)數(shù)種子 BATCH_SIZE = 128 # 批次數(shù)據(jù)大小 LEARNING_RATE = 1e-3 # 學(xué)習(xí)率 EMBEDDING_SIZE = 200 # 詞向量維度 MAX_SENTENCE_SIZE = 50 # 設(shè)置最大語句長度 EPOCH = 20 # 訓(xùn)練測輪次 # 語料路徑 NEG_CORPUS_PATH = ‘。/corpus/neg.txt’ POS_CORPUS_PATH = ‘。/corpus/pos.txt’
utils.en_seg是自定義的文本分詞函數(shù),如下:
def en_seg(sentence): “”“ 簡單的英文分詞方法, :param sentence: 需要分詞的語句 返回分詞結(jié)果 ”“” return sentence.split()
當(dāng)然也可以書寫更復(fù)雜的,或者使用spacy。下面就是書寫讀取文本數(shù)據(jù)到torchtext對象的數(shù)據(jù)了,便于使用torchtext中的方法,如下:
def get_dataset(corpus_path, text_field, label_field, datatype): “”“ 構(gòu)建torchtext數(shù)據(jù)集 :param corpus_path: 數(shù)據(jù)路徑 :param text_field: torchtext設(shè)置的文本域 :param label_field: torchtext設(shè)置的文本標(biāo)簽域 :param datatype: 文本的類別 torchtext格式的數(shù)據(jù)集以及設(shè)置的域 ”“” fields = [(‘text’, text_field), (‘label’, label_field)] examples = [] with open(corpus_path, encoding=‘utf8’) as reader: for line in reader: content = line.rstrip() if datatype == ‘pos’: label = 1 else: label = 0 # content[:-2]是由于原始文本最后的兩個內(nèi)容是空格和。,這里直接去掉,并將數(shù)據(jù)與設(shè)置的域?qū)?yīng)起來 examples.append(data.Example.fromlist([content[:-2], label], fields)) return examples, fields
現(xiàn)在就可以獲取torchtext格式的數(shù)據(jù)了,如下:
# 構(gòu)建data數(shù)據(jù) pos_examples, pos_fields = dataloader.get_dataset(config.POS_CORPUS_PATH, TEXT, LABEL, ‘pos’) neg_examples, neg_fields = dataloader.get_dataset(config.NEG_CORPUS_PATH, TEXT, LABEL, ‘neg’) all_examples, all_fields = pos_examples + neg_examples, pos_fields + neg_fields # 構(gòu)建torchtext類型的數(shù)據(jù)集 total_data = data.Dataset(all_examples, all_fields)
有了上面的數(shù)據(jù),下面就可以快速地為準(zhǔn)備模型需要的數(shù)據(jù)了,如切分,構(gòu)造批次數(shù)據(jù),獲取字典等,如下:
# 數(shù)據(jù)集切分 train_data, test_data = total_data.split(random_state=random.seed(config.RANDOM_SEED), split_ratio=0.8) # 切分后的數(shù)據(jù)查看 # # 數(shù)據(jù)維度查看 print(‘len of train data: %r’ % len(train_data)) # len of train data: 8530 print(‘len of test data: %r’ % len(test_data)) # len of test data: 2132 # # 抽一條數(shù)據(jù)查看 print(train_data.examples[100].text) # [‘never’, ‘engaging’, ‘,’, ‘utterly’, ‘predictable’, ‘a(chǎn)nd’, ‘completely’, ‘void’, ‘of’, ‘a(chǎn)nything’, ‘remotely’, # ‘interesting’, ‘or’, ‘suspenseful’] print(train_data.examples[100].label) # 0 # 為該樣本數(shù)據(jù)構(gòu)建字典,并將子每個單詞映射到對應(yīng)數(shù)字 TEXT.build_vocab(train_data) LABEL.build_vocab(train_data) # 查看字典長度 print(len(TEXT.vocab)) # 19206 # 查看字典中前10個詞語 print(TEXT.vocab.itos[:10]) # [‘《unk》’, ‘《pad》’, ‘,’, ‘the’, ‘a(chǎn)’, ‘a(chǎn)nd’, ‘of’, ‘to’, ‘?!?, ‘is’] # 查找‘name’這個詞對應(yīng)的詞典序號, 本質(zhì)是一個dict print(TEXT.vocab.stoi[‘name’]) # 2063 # 構(gòu)建迭代(iterator)類型的數(shù)據(jù) train_iterator, test_iterator = data.BucketIterator.splits((train_data, test_data), batch_size=config.BATCH_SIZE, sort=False)
這樣一看,是不是減少了我們書寫的很多代碼了。下面就是老生常談的模型預(yù)測和模型效果查看了。
2 構(gòu)建模型并訓(xùn)練
模型的相關(guān)理論已在前文介紹,如果忘了可以回過頭看看。模型還是那個模型,如下:
import torch from torch import nn import config class TextCNN(nn.Module): # output_size為輸出類別(2個類別,0和1),三種kernel,size分別是3,4,5,每種kernel有100個 def __init__(self, vocab_size, embedding_dim, output_size, filter_num=100, kernel_list=(3, 4, 5), dropout=0.5): super(TextCNN, self).__init__() self.embedding = nn.Embedding(vocab_size, embedding_dim) # 1表示channel_num,filter_num即輸出數(shù)據(jù)通道數(shù),卷積核大小為(kernel, embedding_dim) self.convs = nn.ModuleList([ nn.Sequential(nn.Conv2d(1, filter_num, (kernel, embedding_dim)), nn.LeakyReLU(), nn.MaxPool2d((config.MAX_SENTENCE_SIZE - kernel + 1, 1))) for kernel in kernel_list ]) self.fc = nn.Linear(filter_num * len(kernel_list), output_size) self.dropout = nn.Dropout(dropout) def forward(self, x): x = self.embedding(x) # [128, 50, 200] (batch, seq_len, embedding_dim) x = x.unsqueeze(1) # [128, 1, 50, 200] 即(batch, channel_num, seq_len, embedding_dim) out = [conv(x) for conv in self.convs] out = torch.cat(out, dim=1) # [128, 300, 1, 1],各通道的數(shù)據(jù)拼接在一起 out = out.view(x.size(0), -1) # 展平 out = self.dropout(out) # 構(gòu)建dropout層 logits = self.fc(out) # 結(jié)果輸出[128, 2] return logits
為了方便模型訓(xùn)練,測試書寫了兩個函數(shù),當(dāng)然也和之前的相同,如下:
def binary_acc(pred, y): “”“ 計(jì)算模型的準(zhǔn)確率 :param pred: 預(yù)測值 :param y: 實(shí)際真實(shí)值 返回準(zhǔn)確率 ”“” correct = torch.eq(pred, y).float() acc = correct.sum() / len(correct) return acc def train(model, train_data, optimizer, criterion): “”“ 模型訓(xùn)練 :param model: 訓(xùn)練的模型 :param train_data: 訓(xùn)練數(shù)據(jù) :param optimizer: 優(yōu)化器 :param criterion: 損失函數(shù) 該論訓(xùn)練各批次正確率平均值 ”“” avg_acc = [] model.train() # 進(jìn)入訓(xùn)練模式 for i, batch in enumerate(train_data): pred = model(batch.text) loss = criterion(pred, batch.label.long()) acc = binary_acc(torch.max(pred, dim=1)[1], batch.label) avg_acc.append(acc) optimizer.zero_grad() loss.backward() optimizer.step() # 計(jì)算所有批次數(shù)據(jù)的結(jié)果 avg_acc = np.array(avg_acc).mean() return avg_acc def evaluate(model, test_data): “”“ 使用測試數(shù)據(jù)評估模型 :param model: 模型 :param test_data: 測試數(shù)據(jù) 該論訓(xùn)練好的模型預(yù)測測試數(shù)據(jù),查看預(yù)測情況 ”“” avg_acc = [] model.eval() # 進(jìn)入測試模式 with torch.no_grad(): for i, batch in enumerate(test_data): pred = model(batch.text) acc = binary_acc(torch.max(pred, dim=1)[1], batch.label) avg_acc.append(acc) return np.array(avg_acc).mean()
涉及相關(guān)包的話,就自行導(dǎo)入即可。下面就是創(chuàng)建模型和模型訓(xùn)練測試了。好緊張,又到了這個環(huán)節(jié)了。
# 創(chuàng)建模型 text_cnn = model.TextCNN(len(TEXT.vocab), config.EMBEDDING_SIZE, len(LABEL.vocab)) # 選取優(yōu)化器 optimizer = optim.Adam(text_cnn.parameters(), lr=config.LEARNING_RATE) # 選取損失函數(shù) criterion = nn.CrossEntropyLoss() # 繪制結(jié)果 model_train_acc, model_test_acc = [], [] # 模型訓(xùn)練 for epoch in range(config.EPOCH): train_acc = utils.train(text_cnn, train_iterator, optimizer, criterion) print(“epoch = {}, 訓(xùn)練準(zhǔn)確率={}”.format(epoch + 1, train_acc)) test_acc = utils.evaluate(text_cnn, test_iterator) print(“epoch = {}, 測試準(zhǔn)確率={}”.format(epoch + 1, test_acc)) model_train_acc.append(train_acc) model_test_acc.append(test_acc) # 繪制訓(xùn)練過程 plt.plot(model_train_acc) plt.plot(model_test_acc) plt.ylim(ymin=0.5, ymax=1.01) plt.title(“The accuracy of textCNN mode”) plt.legend([‘train’, ‘test’]) plt.show()
模型最后的結(jié)果如下:
模型訓(xùn)練過程
這個和之前結(jié)果沒多大區(qū)別,但是在數(shù)據(jù)處理中卻省去更多的時間,并且也更加規(guī)范化。所以還是有時間學(xué)習(xí)一下torchtext咯。
3 總結(jié)
torchtext支持的自然語言處理處理任務(wù)還是比較多的,并且自身還帶有一些數(shù)據(jù)集。最近還在做實(shí)體識別任務(wù),使用的算法模型是bi-lstm+crf。這個任務(wù)的本質(zhì)就是序列標(biāo)注,torchtext也是支持這種類型數(shù)據(jù)的處理的,后期有時間的話也會做相關(guān)的介紹,記得關(guān)注哦。對啦,本文的全部代碼和語料,我都上傳到github上了:https://github.com/Htring/NLP_Applications[4],后續(xù)其他相關(guān)應(yīng)用代碼也會陸續(xù)更新,也歡迎star,指點(diǎn)哦。
原文標(biāo)題:textCNN論文與原理——短文本分類(基于pytorch和torchtext)
文章出處:【微信公眾號:自然語言處理愛好者】歡迎添加關(guān)注!文章轉(zhuǎn)載請注明出處。
責(zé)任編輯:haq
-
python
+關(guān)注
關(guān)注
55文章
4768瀏覽量
84376 -
深度學(xué)習(xí)
+關(guān)注
關(guān)注
73文章
5465瀏覽量
120891
原文標(biāo)題:textCNN論文與原理——短文本分類(基于pytorch和torchtext)
文章出處:【微信號:NLP_lover,微信公眾號:自然語言處理愛好者】歡迎添加關(guān)注!文章轉(zhuǎn)載請注明出處。
發(fā)布評論請先 登錄
相關(guān)推薦
使用LLM進(jìn)行自然語言處理的優(yōu)缺點(diǎn)
RK3588 技術(shù)分享 | 在Android系統(tǒng)中使用NPU實(shí)現(xiàn)Yolov5分類檢測
RK3588 技術(shù)分享 | 在Android系統(tǒng)中使用NPU實(shí)現(xiàn)Yolov5分類檢測
利用TensorFlow實(shí)現(xiàn)基于深度神經(jīng)網(wǎng)絡(luò)的文本分類模型
llm模型有哪些格式
llm模型和chatGPT的區(qū)別
卷積神經(jīng)網(wǎng)絡(luò)在文本分類領(lǐng)域的應(yīng)用
交換機(jī)的基本分類
鴻蒙UI界面——@ohos.measure (文本計(jì)算)
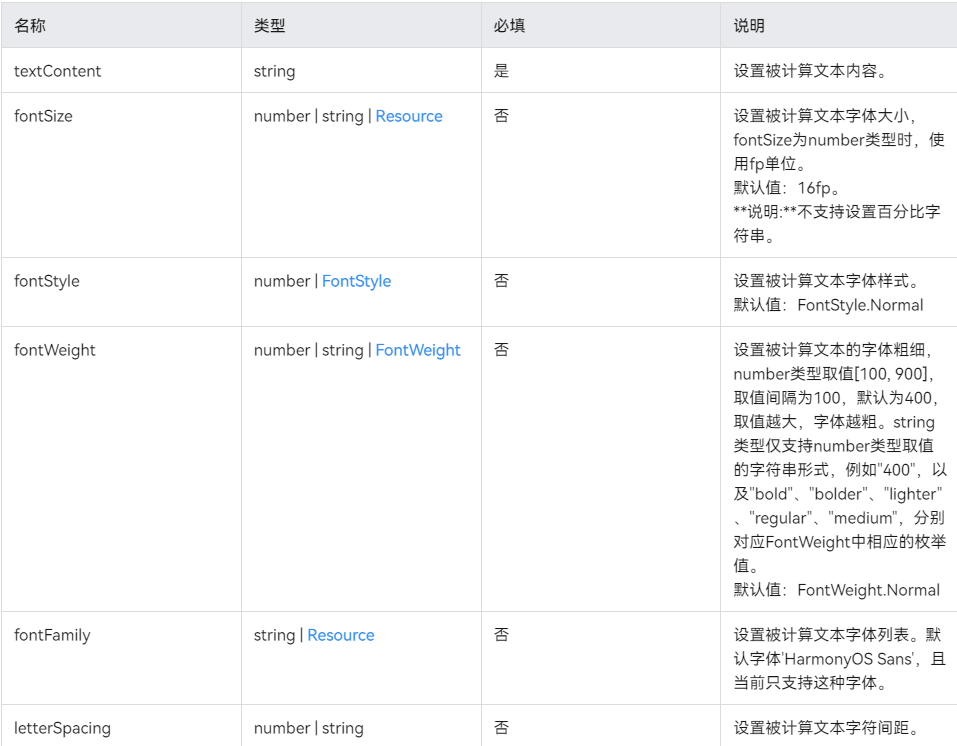
了解如何使用PyTorch構(gòu)建圖神經(jīng)網(wǎng)絡(luò)
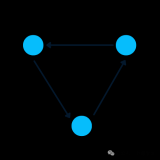
人工智能中文本分類的基本原理和關(guān)鍵技術(shù)
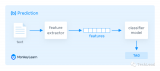
評論