使用到的庫:dlib+Opencvpython版本:3.8編譯環(huán)境:Jupyter Notebook (Anaconda3)
0.Dlib人臉特征檢測原理
提取特征點(diǎn):請參考
首選抓取多張圖片,從中獲取特征數(shù)據(jù)集和平均特征值然后寫入csv文件 - 計(jì)算特征數(shù)據(jù)集的歐式距離作對比:首先使用Opencv庫將攝像頭中的人臉框出來,再將攝像頭中采取到的人臉特征值與數(shù)據(jù)集中的每個(gè)人的特征均值作對比,選取最接近(歐氏距離最?。┑闹?,將其標(biāo)注為歐氏距離最小的數(shù)據(jù)集的人名
一、構(gòu)建人臉特征數(shù)據(jù)集
1、安裝Dlib
請參考
2、構(gòu)建自己的數(shù)據(jù)集
2.1 抓取人臉圖片
在視頻流中抓取人臉特征,并保存為256*256大小的圖片文件共20張,這就是我們建立數(shù)據(jù)集的第一步,用來訓(xùn)練人臉識(shí)別。
“不一定是256*256的尺寸,可以根據(jù)自己的需求來調(diào)整大小,圖片越大訓(xùn)練結(jié)果會(huì)愈加精確,但也會(huì)影響訓(xùn)練模型的時(shí)間。
其中:
光線:曝光和黑暗的圖片需手動(dòng)剔除- 請使用同一個(gè)設(shè)備進(jìn)行數(shù)據(jù)采集,不同設(shè)備的攝像頭采集到的數(shù)據(jù)集會(huì)有出入- 這里采用的是從視頻流中進(jìn)行捕捉截圖,也可以自己準(zhǔn)備20張左右的人臉圖片
代碼:
importcv2 importdlib importos importsys importrandom #存儲(chǔ)位置 output_dir='D:/No1WorkSpace/JupyterNotebook/Facetrainset/Num&Name'#這里填編號+人名 size=256#圖片邊長 ifnotos.path.exists(output_dir): os.makedirs(output_dir) #改變圖片的亮度與對比度 defrelight(img,light=1,bias=0): w=img.shape[1] h=img.shape[0] #image=[] foriinrange(0,w): forjinrange(0,h): forcinrange(3): tmp=int(img[j,i,c]*light+bias) iftmp>255: tmp=255 eliftmp<0: tmp=0 img[j,i,c]=tmp returnimg #使用dlib自帶的frontal_face_detector作為我們的特征提取器 detector=dlib.get_frontal_face_detector() #打開攝像頭參數(shù)為輸入流,可以為攝像頭或視頻文件 camera=cv2.VideoCapture(0) #camera=cv2.VideoCapture('C:/Users/CUNGU/Videos/Captures/wang.mp4') index=1 whileTrue: if(index<=20):#存儲(chǔ)15張人臉特征圖像 print('Beingprocessedpicture%s'%index) #從攝像頭讀取照片 success,img=camera.read() #轉(zhuǎn)為灰度圖片 gray_img=cv2.cvtColor(img,cv2.COLOR_BGR2GRAY) #使用detector進(jìn)行人臉檢測 dets=detector(gray_img,1) fori,dinenumerate(dets): x1=d.top()ifd.top()>0else0 y1=d.bottom()ifd.bottom()>0else0 x2=d.left()ifd.left()>0else0 y2=d.right()ifd.right()>0else0 face=img[x1:y1,x2:y2] #調(diào)整圖片的對比度與亮度,對比度與亮度值都取隨機(jī)數(shù),這樣能增加樣本的多樣性 face=relight(face,random.uniform(0.5,1.5),random.randint(-50,50)) face=cv2.resize(face,(size,size)) cv2.imshow('image',face) cv2.imwrite(output_dir+'/'+str(index)+'.jpg',face) index+=1 key=cv2.waitKey(30)&0xff ifkey==27: break else: print('Finished!') #釋放攝像頭releasecamera camera.release() #刪除建立的窗口deleteallthewindows cv2.destroyAllWindows() break
運(yùn)行效果:
2.2 分析每張人臉的特征值并存入csv文件
根據(jù)抓取的圖片和人臉識(shí)別模型->訓(xùn)練得到的20個(gè)的68個(gè)特征數(shù)據(jù)集以及1個(gè)平均特征值存入csv文件
“每張圖片的68個(gè)特征數(shù)據(jù)集可以不用存取,他們只是中間量,計(jì)算平均值以后就可以拋棄了,這里把他們輸出出來只是為了方便學(xué)習(xí)。
代碼:
#從人臉圖像文件中提取人臉特征存入CSV #Featuresextractionfromimagesandsaveintofeatures_all.csv #return_128d_features()獲取某張圖像的128D特征 #compute_the_mean()計(jì)算128D特征均值 fromcv2importcv2ascv2 importos importdlib fromskimageimportio importcsv importnumpyasnp #要讀取人臉圖像文件的路徑 path_images_from_camera="D:/No1WorkSpace/JupyterNotebook/Facetrainset/" #Dlib正向人臉檢測器 detector=dlib.get_frontal_face_detector() #Dlib人臉預(yù)測器 predictor=dlib.shape_predictor("D:/No1WorkSpace/JupyterNotebook/model/shape_predictor_68_face_landmarks.dat") #Dlib人臉識(shí)別模型 #Facerecognitionmodel,theobjectmapshumanfacesinto128Dvectors face_rec=dlib.face_recognition_model_v1("D:/No1WorkSpace/JupyterNotebook/model/dlib_face_recognition_resnet_model_v1.dat") #返回單張圖像的128D特征 defreturn_128d_features(path_img): img_rd=io.imread(path_img) img_gray=cv2.cvtColor(img_rd,cv2.COLOR_BGR2RGB) faces=detector(img_gray,1) print("%-40s%-20s"%("檢測到人臉的圖像/imagewithfacesdetected:",path_img),' ') #因?yàn)橛锌赡芙叵聛淼娜四樤偃z測,檢測不出來人臉了 #所以要確保是檢測到人臉的人臉圖像拿去算特征 iflen(faces)!=0: shape=predictor(img_gray,faces[0]) face_descriptor=face_rec.compute_face_descriptor(img_gray,shape) else: face_descriptor=0 print("noface") returnface_descriptor #將文件夾中照片特征提取出來,寫入CSV defreturn_features_mean_personX(path_faces_personX): features_list_personX=[] photos_list=os.listdir(path_faces_personX) ifphotos_list: foriinrange(len(photos_list)): withopen("D:/No1WorkSpace/JupyterNotebook/feature/featuresGiao"+str(i)+".csv","w",newline="")ascsvfile: writer=csv.writer(csvfile) #調(diào)用return_128d_features()得到128d特征 print("%-40s%-20s"%("正在讀的人臉圖像/imagetoread:",path_faces_personX+"/"+photos_list[i])) features_128d=return_128d_features(path_faces_personX+"/"+photos_list[i]) print(features_128d) writer.writerow(features_128d) #遇到?jīng)]有檢測出人臉的圖片跳過 iffeatures_128d==0: i+=1 else: features_list_personX.append(features_128d) else: print("文件夾內(nèi)圖像文件為空/Warning:Noimagesin"+path_faces_personX+'/',' ') #計(jì)算128D特征的均值 #Nx128D->1x128D iffeatures_list_personX: features_mean_personX=np.array(features_list_personX).mean(axis=0) else: features_mean_personX='0' returnfeatures_mean_personX #讀取某人所有的人臉圖像的數(shù)據(jù) people=os.listdir(path_images_from_camera) people.sort() withopen("D:/No1WorkSpace/JupyterNotebook/feature/features_all.csv","w",newline="")ascsvfile: writer=csv.writer(csvfile) forpersoninpeople: print("#####"+person+"#####") #Getthemean/averagefeaturesofface/personX,itwillbealistwithalengthof128D features_mean_personX=return_features_mean_personX(path_images_from_camera+person) writer.writerow(features_mean_personX) print("特征均值/Themeanoffeatures:",list(features_mean_personX)) print(' ') print("所有錄入人臉數(shù)據(jù)存入/Saveallthefeaturesoffacesregisteredinto:D:/myworkspace/JupyterNotebook/People/feature/features_all2.csv")
“如果要輸出每一張圖片的特征數(shù)據(jù)集,這里要用到Python的文件批量生成。
代碼運(yùn)行效果
二、識(shí)別人臉并匹配數(shù)據(jù)集
1、原理:
通過計(jì)算特征數(shù)據(jù)集的歐氏距離作對比來識(shí)別人臉,取歐氏距離最小的數(shù)據(jù)集進(jìn)行匹配。
“歐氏距離也稱歐幾里得距離或歐幾里得度量,是一個(gè)通常采用的距離定義,它是在m維空間中兩個(gè)點(diǎn)之間的真實(shí)距離。在二維和三維空間中的歐氏距離的就是兩點(diǎn)之間的距離。使用這個(gè)距離,歐氏空間成為度量空間。相關(guān)聯(lián)的范數(shù)稱為歐幾里得范數(shù)。較早的文獻(xiàn)稱之為畢達(dá)哥拉斯度量。二維空間公式:
2、視頻流實(shí)時(shí)識(shí)別人臉數(shù)據(jù)
代碼:
#攝像頭實(shí)時(shí)人臉識(shí)別 importos importdlib#人臉處理的庫Dlib importcsv#存入表格 importtime importsys importnumpyasnp#數(shù)據(jù)處理的庫numpy fromcv2importcv2ascv2#圖像處理的庫OpenCv importpandasaspd#數(shù)據(jù)處理的庫Pandas #人臉識(shí)別模型,提取128D的特征矢量 #facerecognitionmodel,theobjectmapshumanfacesinto128Dvectors #Referthistutorial:http://dlib.net/python/index.html#dlib.face_recognition_model_v1 facerec=dlib.face_recognition_model_v1("D:/No1WorkSpace/JupyterNotebook/model/dlib_face_recognition_resnet_model_v1.dat") #計(jì)算兩個(gè)128D向量間的歐式距離 #computethee-distancebetweentwo128Dfeatures defreturn_euclidean_distance(feature_1,feature_2): feature_1=np.array(feature_1) feature_2=np.array(feature_2) dist=np.sqrt(np.sum(np.square(feature_1-feature_2))) returndist #處理存放所有人臉特征的csv path_features_known_csv="D:/No1WorkSpace/JupyterNotebook/feature/features_all.csv" csv_rd=pd.read_csv(path_features_known_csv,header=None) #用來存放所有錄入人臉特征的數(shù)組 #thearraytosavethefeaturesoffacesinthedatabase features_known_arr=[] #讀取已知人臉數(shù)據(jù) #printknownfaces foriinrange(csv_rd.shape[0]): features_someone_arr=[] forjinrange(0,len(csv_rd.loc[i,:])): features_someone_arr.append(csv_rd.loc[i,:][j]) features_known_arr.append(features_someone_arr) print("Faces in Database:",len(features_known_arr)) #Dlib檢測器和預(yù)測器 #Thedetectorandpredictorwillbeused detector=dlib.get_frontal_face_detector() predictor=dlib.shape_predictor('D:/No1WorkSpace/JupyterNotebook/model/shape_predictor_68_face_landmarks.dat') #創(chuàng)建cv2攝像頭對象 #cv2.VideoCapture(0)tousethedefaultcameraofPC, #andyoucanuselocalvideonamebyusecv2.VideoCapture(filename) cap=cv2.VideoCapture(0) #cap.set(propId,value) #設(shè)置視頻參數(shù),propId設(shè)置的視頻參數(shù),value設(shè)置的參數(shù)值 cap.set(3,480) #cap.isOpened()返回true/false檢查初始化是否成功 #whenthecameraisopen whilecap.isOpened(): flag,img_rd=cap.read() kk=cv2.waitKey(1) #取灰度 img_gray=cv2.cvtColor(img_rd,cv2.COLOR_RGB2GRAY) #人臉數(shù)faces faces=detector(img_gray,0) #待會(huì)要寫的字體fonttowritelater font=cv2.FONT_HERSHEY_COMPLEX #存儲(chǔ)當(dāng)前攝像頭中捕獲到的所有人臉的坐標(biāo)/名字 #thelisttosavethepositionsandnamesofcurrentfacescaptured pos_namelist=[] name_namelist=[] #按下q鍵退出 #press'q'toexit ifkk==ord('q'): break else: #檢測到人臉whenfacedetected iflen(faces)!=0: #獲取當(dāng)前捕獲到的圖像的所有人臉的特征,存儲(chǔ)到features_cap_arr #getthefeaturescapturedandsaveintofeatures_cap_arr features_cap_arr=[] foriinrange(len(faces)): shape=predictor(img_rd,faces[i]) features_cap_arr.append(facerec.compute_face_descriptor(img_rd,shape)) #遍歷捕獲到的圖像中所有的人臉 #traversalallthefacesinthedatabase forkinrange(len(faces)): print("#####cameraperson",k+1,"#####") #讓人名跟隨在矩形框的下方 #確定人名的位置坐標(biāo) #先默認(rèn)所有人不認(rèn)識(shí),是unknown #setthedefaultnamesoffaceswith"unknown" name_namelist.append("unknown") #每個(gè)捕獲人臉的名字坐標(biāo)thepositionsoffacescaptured pos_namelist.append(tuple([faces[k].left(),int(faces[k].bottom()+(faces[k].bottom()-faces[k].top())/4)])) #對于某張人臉,遍歷所有存儲(chǔ)的人臉特征 #foreveryfacesdetected,comparethefacesinthedatabase e_distance_list=[] foriinrange(len(features_known_arr)): #如果person_X數(shù)據(jù)不為空 ifstr(features_known_arr[i][0])!='0.0': print("withperson",str(i+1),"theedistance:",end='') e_distance_tmp=return_euclidean_distance(features_cap_arr[k],features_known_arr[i]) print(e_distance_tmp) e_distance_list.append(e_distance_tmp) else: #空數(shù)據(jù)person_X e_distance_list.append(999999999) #找出最接近的一個(gè)人臉數(shù)據(jù)是第幾個(gè) #Findtheonewithminimumedistance similar_person_num=e_distance_list.index(min(e_distance_list)) print("Minimumedistancewithperson",int(similar_person_num)+1) #計(jì)算人臉識(shí)別特征與數(shù)據(jù)集特征的歐氏距離 #距離小于0.4則標(biāo)出為可識(shí)別人物 ifmin(e_distance_list)<0.4: #這里可以修改攝像頭中標(biāo)出的人名 #Hereyoucanmodifythenamesshownonthecamera #1、遍歷文件夾目錄 folder_name='D:/No1WorkSpace/JupyterNotebook/Facetrainset/' #最接近的人臉 sum=similar_person_num+1 key_id=1#從第一個(gè)人臉數(shù)據(jù)文件夾進(jìn)行對比 #獲取文件夾中的文件名:1wang、2zhou、3... file_names=os.listdir(folder_name) fornameinfile_names: #print(name+'->'+str(key_id)) ifsum==key_id: #winsound.Beep(300,500)#響鈴:300頻率,500持續(xù)時(shí)間 name_namelist[k]=name[1:]#人名刪去第一個(gè)數(shù)字(用于視頻輸出標(biāo)識(shí)) key_id+=1 #播放歡迎光臨音效 #playsound('D:/myworkspace/JupyterNotebook/People/music/welcome.wav') #print("Maybeperson"+str(int(similar_person_num)+1)) #-----------篩選出人臉并保存到visitor文件夾------------ fori,dinenumerate(faces): x1=d.top()ifd.top()>0else0 y1=d.bottom()ifd.bottom()>0else0 x2=d.left()ifd.left()>0else0 y2=d.right()ifd.right()>0else0 face=img_rd[x1:y1,x2:y2] size=64 face=cv2.resize(face,(size,size)) #要存儲(chǔ)visitor人臉圖像文件的路徑 path_visitors_save_dir="D:/No1WorkSpace/JupyterNotebook/KnownFacetrainset/" #存儲(chǔ)格式:2019-06-24-14-33-40wang.jpg now_time=time.strftime("%Y-%m-%d-%H-%M-%S",time.localtime()) save_name=str(now_time)+str(name_namelist[k])+'.jpg' #print(save_name) #本次圖片保存的完整url save_path=path_visitors_save_dir+'/'+save_name #遍歷visitor文件夾所有文件名 visitor_names=os.listdir(path_visitors_save_dir) visitor_name='' fornameinvisitor_names: #名字切片到分鐘數(shù):2019-06-26-11-33-00wangyu.jpg visitor_name=(name[0:16]+'-00'+name[19:]) #print(visitor_name) visitor_save=(save_name[0:16]+'-00'+save_name[19:]) #print(visitor_save) #一分鐘之內(nèi)重復(fù)的人名不保存 ifvisitor_save!=visitor_name: cv2.imwrite(save_path,face) print('新存儲(chǔ):'+path_visitors_save_dir+'/'+str(now_time)+str(name_namelist[k])+'.jpg') else: print('重復(fù),未保存!') else: #播放無法識(shí)別音效 #playsound('D:/myworkspace/JupyterNotebook/People/music/sorry.wav') print("Unknownperson") #-----保存圖片------- #-----------篩選出人臉并保存到visitor文件夾------------ fori,dinenumerate(faces): x1=d.top()ifd.top()>0else0 y1=d.bottom()ifd.bottom()>0else0 x2=d.left()ifd.left()>0else0 y2=d.right()ifd.right()>0else0 face=img_rd[x1:y1,x2:y2] size=64 face=cv2.resize(face,(size,size)) #要存儲(chǔ)visitor-》unknown人臉圖像文件的路徑 path_visitors_save_dir="D:/No1WorkSpace/JupyterNotebook/UnKnownFacetrainset/" #存儲(chǔ)格式:2019-06-24-14-33-40unknown.jpg now_time=time.strftime("%Y-%m-%d-%H-%M-%S",time.localtime()) #print(save_name) #本次圖片保存的完整url save_path=path_visitors_save_dir+'/'+str(now_time)+'unknown.jpg' cv2.imwrite(save_path,face) print('新存儲(chǔ):'+path_visitors_save_dir+'/'+str(now_time)+'unknown.jpg') #矩形框 #drawrectangle forkk,dinenumerate(faces): #繪制矩形框 cv2.rectangle(img_rd,tuple([d.left(),d.top()]),tuple([d.right(),d.bottom()]),(0,255,255),2) print(' ') #在人臉框下面寫人臉名字 #writenamesunderrectangle foriinrange(len(faces)): cv2.putText(img_rd,name_namelist[i],pos_namelist[i],font,0.8,(0,255,255),1,cv2.LINE_AA) print("Facesincameranow:",name_namelist," ") #cv2.putText(img_rd,"Press'q':Quit",(20,450),font,0.8,(84,255,159),1,cv2.LINE_AA) cv2.putText(img_rd,"FaceRecognition",(20,40),font,1,(0,0,255),1,cv2.LINE_AA) cv2.putText(img_rd,"Visitors:"+str(len(faces)),(20,100),font,1,(0,0,255),1,cv2.LINE_AA) #窗口顯示showwithopencv cv2.imshow("camera",img_rd) #釋放攝像頭releasecamera cap.release() #刪除建立的窗口deleteallthewindows cv2.destroyAllWindows()
“若直接使用本代碼,文件目錄弄成中文會(huì)亂碼
圖中兩人的特征數(shù)據(jù)集均已被收集并錄入,所以可以識(shí)別出來,如果沒有被錄入的人臉就會(huì)出現(xiàn)unknown。
-
攝像頭
+關(guān)注
關(guān)注
59文章
4754瀏覽量
94410 -
編譯
+關(guān)注
關(guān)注
0文章
646瀏覽量
32673 -
OpenCV
+關(guān)注
關(guān)注
29文章
622瀏覽量
41093
原文標(biāo)題:手把手教你運(yùn)用Python實(shí)現(xiàn)進(jìn)階版人臉識(shí)別
文章出處:【微信號:vision263com,微信公眾號:新機(jī)器視覺】歡迎添加關(guān)注!文章轉(zhuǎn)載請注明出處。
發(fā)布評論請先 登錄
相關(guān)推薦
在Python中利用dlib進(jìn)行人臉檢測
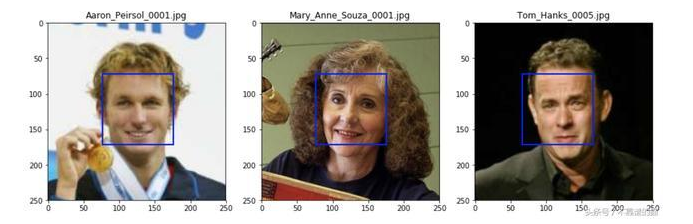
評論